- Samer G Karam, doctoral student1 2,
- Yuan Zhang, assistant clinical professor1 2,
- Hector Pardo-Hernandez, researcher3 4,
- Uwe Siebert, professor5 6 7,
- Laura Koopman, senior adviser8,
- Jane Noyes, professor9,
- Jean-Eric Tarride, professor1 10 11,
- Adrienne L Stevens, manager12,
- Vivian Welch, senior investigator13,
- Zuleika Saz-Parkinson, project adviser14,
- Brendalynn Ens, director (retired)15,
- Tahira Devji, medical student16,
- Feng Xie, professor1 10,
- Glen Hazlewood, associate professor17 18,
- Lawrence Mbuagbaw, associate professor1 19 20 21 22 23,
- Pablo Alonso-Coello, senior researcher3 4 24,
- Jan L Brozek, associate professor1 2,
- Holger J Schünemann, professor1 25
1Department of Health Research Methods, Evidence and Impact, McMaster University, Hamilton, ON, Canada
2Michael G DeGroote Cochrane Canada and McMaster GRADE Centres, McMaster University, Hamilton, ON, Canada
3Iberoamerican Cochrane Centre, Sant Antoni Maria Claret, Barcelona, Spain
4Centro de Investigación Biomédica en Red de Epidemiología y Salud Pública (CIBERESP), Madrid, Spain
5Department of Public Health, Health Services Research and Health Technology Assessment, Institute of Public Health, Medical Decision Making and Health Technology Assessment, UMIT TIROL-University for Health Sciences and Technology, Hall in Tirol, Austria
6Center for Health Decision Science and Departments of Epidemiology and Health Policy and Management, Harvard T H Chan School of Public Health, Boston, MA, USA
7Institute for Technology Assessment and Department of Radiology, Massachusetts General Hospital, Harvard Medical School, Boston, MA, USA
8Department of Specialist Medical Care, National Health Care Institute, Diemen, Netherlands
9School of Medical and Health Sciences, Bangor University, Wales, UK
10Centre for Health Economics and Policy Analysis, McMaster University Faculty of Health Sciences, Hamilton, ON, Canada
11Programs for Assessment of Technologies in Health, St Joseph’s Healthcare Hamilton, Hamilton, ON, Canada
12Centre for Immunisation Programmes, Public Health Agency of Canada, ON, Canada
13Bruyère Research Institute and, School of Epidemiology and Public Health, University of Ottawa, Ottawa, ON, Canada
14European Commission, Joint Research Centre, Ispra, Italy
15Implementation Support and Knowledge Mobilisation, Canadian Agency for Drugs and Technologies in Health, Ottawa, ON, Canada
16Temerty Faculty of Medicine, University of Toronto, Toronto, ON, Canada.
17Department of Medicine, Cumming School of Medicine, University of Calgary, Calgary, AB, Canada
18Department of Community Health Sciences, Cumming School of Medicine, University of Calgary, Calgary, AB, Canada
19Department of Anaesthesia, McMaster University, Hamilton, ON, Canada
20Department of Paediatrics, McMaster University, Hamilton, ON, Canada
21Biostatistics Unit, Father Sean O’Sullivan Research Centre, St Joseph’s Healthcare, Hamilton, ON, Canada
22Centre for Development of Best Practices in Health, Yaoundé Central Hospital, Yaoundé, Cameroon
23Division of Epidemiology and Biostatistics, Department of Global Health, Stellenbosch University, Cape Town, South Africa
24Institut de Recerca Sant Pau (IR SANT PAU), Sant Quintí, Barcelona, Spain
25Clinical Epidemiology and Research Centre (CERC), Humanitas Universityand Humanitas Research Hospital, Via Rita Levi Montalcini 4, 20090 Pieve Emanuele, Milan, Italy
- Correspondence to: H J Schünemann schuneh{at}mcmaster.ca
Healthcare decision making relies on evidence on the relative effectiveness, safety, and cost effectiveness of an intervention evaluated in appropriate studies.12 Choosing between different interventions (such as preventive, diagnostic, or treatment strategies) depends on the importance or value that people place on specific health states or health outcomes.2 Values have a major role at different levels of decision making, from the individual level to the healthcare system level. In this context, people’s values reflect the importance they place on outcomes of interest that result from decisions about using an intervention—for example, taking a certain test or starting a new treatment regimen.2 We use the term “people” when talking about value because the term is inclusive to patients, healthcare providers, policy makers, and the general public.
Utility instruments are widely used to elicit the absolute value of a health outcome, and provide an index measure anchored on a scale with 1 reflecting perfect health and 0 reflecting being dead.34 Various methods are used to establish values, including direct measures of utility, indirect measurements of utility, or qualitative research.25 The visual analogue scale (VAS) is one of the simplest measures to elicit these values. People are asked to rate a health state on a VAS that is then converted to a utility value.67 While the scale directly measures the importance of an outcome, concerns exist about how accurate and valid it might be.2 Other direct measures such as the standard gamble and time trade-off require people to choose between their current health state and a treatment option that could result in perfect health or in immediate death.48 Discrete choice experiments ask people to choose between two or more treatment options where the choices differ in terms of their attributes, that are defined by the investigators.9 The relative importance of each attribute is then inferred by analysing the responses, assuming that patients choose the option with the highest value.9 Indirect methods of measuring utility values include validated, health related, quality-of-life instruments, such as the EQ-5D and the Health Utilities Index.10 The EQ-5D requires respondents to answer questions across five domains that are converted to a utility value using validated scoring systems.1112
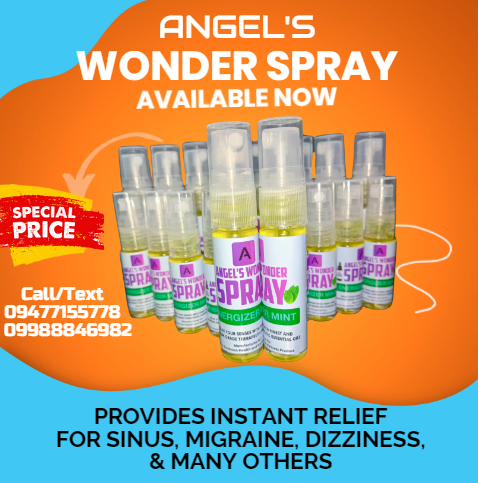
Summary points
-
Assessing the risk of bias in individual studies is an essential step to determine overall certainty of evidence in a systematic review or health technology assessment and for guideline development
-
The Risk of Bias in Values and Utilities (ROBVALU) tool assesses risk of bias in quantitative studies of people’s values, utilities, or importance of outcomes
-
A sequential mixed methods approach was used to develop ROBVALU, initially based on signalling questions and subdomains developed by the GRADE working group to assess risk of bias; a modified Delphi approach was used for final refinement of the tool
-
ROBVALU covers four separate subdomains through which bias might be introduced; individual subdomain judgments inform the overall risk of bias of studies
-
ROBVALU has demonstrated high validity and reliability
General application of utility values in research
These utility values allow researchers to weigh the benefits and harms of an option and, thus, they also are important in health economics and health technology assessments.313 For instance, in decision analysis, they are required to calculate quality adjusted life years. Confidence in studies that report on values needs to be ascertained for decision making in guideline recommendations, health technology assessments, or coverage decision.14 For example, in a systematic review on people with chronic obstructive pulmonary disease, we found moderate certainty that patients value adverse events as important, but on average valued them as less important than symptom relief.15 We also found moderate certainty that exacerbation and hospital admission owing to exacerbation are the outcomes that patients with chronic obstructive pulmonary disease rate as most important. In another example, a systematic review on patients’ values on venous thromboembolism, we found that people with cancer placed more importance on a reduction in new or recurrent venous thromboembolism than on a decrease in major or minor bleeding events.16
The Grading of Recommendations, Assessment, Development, and Evaluation (GRADE) Evidence to Decision frameworks is a widely used approach in guidelines, health technology assessment, and other decisions. The frameworks require judgments about the certainty in how much people value the main outcomes: “Is there important uncertainty about . . . how much people value the main outcomes?”1718 A key determinant of certainty is internal validity—that is, how well individual studies were designed and conducted (ie, internal validity, which GRADE and Cochrane label as the risk-of-bias (ROB) domain).
Risk of bias
Similar to other study designs, threats to internal validity arising from the study design, conduct, analysis, and reporting of the study introduce ROB in research on utility values.2 Poor study quality could result in indirectness which encompasses applicability and external validity, often as a result of PICO (patient/population, intervention, comparison, and outcomes) elements. Another quality issue is low sample size or no sample size calculation, which could result in imprecision. ROB assessment tools are developed to assess biases that result in threats of internal validity and would not measure indirectness and precision. Quality assessment tools and reporting checklists often include all factors of a study’s qualities and safeguards, but these tools differ from a ROB assessment tool that aims to present a ROB judgment for a study. A key factor that might introduce bias in values studies is the instrument used to measure utilities of the people in the study. Bias means that a value people place on an outcome in a research study (eg, a value of 0.5 for stroke) would be systematically different from the true value that people would place on that outcome. For example, the true unbiased value might be 0.3 and, thus, use of biased estimates would provide inaccurate answers in the modelling and health decision making context.
ROB assessment tools exist for many study designs, including the Cochrane Risk of Bias 2 (RoB 2) for randomised trials,19 ROBINS-I for non-randomised studies of the effects of interventions,20 and ROBINS-E for studies about exposures.2122 Critical appraisal tools to assess the quality of a study are also study design specific, such as the Newcastle-Ottawa scale and the Joanna Briggs Institute’s critical appraisal tool for cross sectional studies.2324 These tools are regularly used by researchers to assess the quality of individual studies or to assess ROB, but they were not developed for studies on utility values. These checklists invariably include questions specific to the study design, which would not always be appropriate to answer in studies about people’s values (eg, “Were there deviations from the intended intervention that arose because of the trial context?” or “Was the exposure measured in a valid and reliable way?”).192122 For studies on utility values, a major concern that is not adequately addressed by any commonly used ROB tool is the method used to elicit people’s values. The measurement instrument needs to be valid and reliable, be used appropriately, use valid health outcomes, and explore proper understanding of the instrument. No validated tool is available for the nuanced assessment of ROB in individual studies measuring utility values.9202526
Objective
To properly implement evidence based decision making and formulate evidence based recommendations in clinical or public health guidelines, evaluation of ROB is crucial in studies of values, utilities, or importance of outcomes. However, owing to the absence of specialised and validated tools to assess ROB, this evaluation is rarely done. Thus, our goal was to develop, validate, and describe a pragmatic tool for studies measuring the value people place on health outcomes with appropriate guidance to apply it correctly.
Development of the ROBVALU tool and guidance
We used a sequential, mixed methods approach to develop ROBVALU and related guidance document (supplement S1),27 starting with a qualitative approach and followed by a quantitative phase to assess the psychometric properties of the tool (fig 1). In the qualitative phase, we first considered the ROB signalling questions (appendix table A1) and subdomains that we had developed for GRADE guidance to assess ROB about values across studies in a body of evidence.2 For that GRADE guidance, we iteratively developed the subdomains and signalling questions starting with a list of 23 items identified as part of a systematic survey project.26 The core research group reviewed the 23 items to identify any missing item that might be relevant for the single study ROBVALU tool. After thorough group discussions, a decision was made not to add any new items or subdomains to avoid complexity, thereby improving applicability, feasibility, and adoption of the tool.
We first structured a preliminary version of the tool and added simple considerations to help answer the signalling questions. These signalling questions were categorised into four subdomains: selection of participants into the study, completeness of data, measurement instrument, and data analysis. We used a 4 point, Likert-type scale (ie, yes, probably yes, probably no, no) to judge the individual items, to avoid a neutral option of a 5 point Likert scale when studies lack sufficient information to make a proper judgment. In each subdomain, the tool asked how important and how serious the ROB issue is. The core research group iteratively revised the tool and accompanying guidance document. An advisory group of experts provided feedback and suggested appropriate changes to establish face and content validity (supplement S2).
Participant testing
We used purposeful sampling to recruit 15 participants with experience in critical appraisal, systematic reviews, or guidelines for user testing and semi-structured interviews (supplement S3). The participants had a broad level of expertise, from masters level students to senior researchers with experience in health research ranging from six months to 30 years (appendix table A2). All users received the ROBVALU tool and the accompanying guidance document (supplement S1). We instructed the participants to complete three to four assessments and every sample study was assessed by four users independently, 11 studies in total were assessed (appendix table A3). Based on feedback received in the semi-structured interview after user testing, we iteratively revised and improved the guidance document throughout the project with a focus on the wordings, spelling, and grammatical structure of the guidance document. The ROBVALU tool demonstrated good psychometric properties with an overall intraclass correlation coefficient of 0.87 and the four subdomains showed good to excellent reliability ranging from 0.80 to 0.91 (table 1 and supplement S4). We also calculated the inter-rater reliability of the global ROB judgment using the ROBVALU tool with Kendall’s W, which showed substantial agreement of 0.62 (supplement S4). We invited four expert participants in the field to provide a global judgment for ROB without using the ROBVALU, with each expert rating three to four studies. When we added expert participant responses of the global ROB judgment, the Kendall’s W dropped to 0.45, showing moderate agreement (supplement S4). However, only four global judgment responses were more than one level of seriousness higher or lower than the expert participant judgment (appendix table A4).
Reliability of the Risk of Bias in Values and Utilities (ROBVALU) tool. CI=confidence interval
Modified Delphi process
Finally, following our protocol, we used purposeful sampling to invite 20 experts in values, utilities, health technology assessment, and health decision science to participate in a modified Delphi process for final refinement of the tool (supplement S5, fig S8).282930 We used our extensive network of global colleagues working in the field of study to identify and invite the expert panel. Ten voting members accepted the invite to participate in the Delphi panel, and four members of the working group participated as non-voting members. We shared the ROBVALU tool draft, guidance document, and results of our participant testing with the panel members.
The first round of the Delphi process involved an anonymous survey to determine the signalling questions to be included. The second round took place via recorded video conferences with the aim of identifying common themes and reaching consensus on simplifying and harmonising language across the tool. The third and final round of the Delphi process included an anonymous survey for final consensus on the wording of the signalling questions and the proposed methods for providing a global ROB judgment. We used Google forms to prepare the surveys; the first survey used a 7 point Likert scale (ie, strongly agree, agree, somewhat agree, neutral, somewhat agree, disagree, and strongly disagree) to rate each item, with 70% agreement set as the cut-off threshold to retain or remove a signalling question. The final survey used a 3 point scale (ie, agree, neutral, and disagree) with a 70% agreement set as the cut-off threshold to retain the signalling question.
We had a 100% response rate in the first round of the Delphi process, with 80-100% consensus to retain all signalling questions. We also collected feedback from open ended questions for suggested edits for the signalling questions (supplement S6). In the second round of the Delphi process, we presented the ROBVALU tool, psychometric properties, exploratory factor analysis, and results of the first round of the Delphi to the panel members. After deliberating on the tool’s properties, agreement was reached to edit some signalling questions to simplify the language or to harmonise the language across the tool, which resulted in minor changes only. We also discussed how to make a final judgment for ROB for a study.
We had a 100% response rate in the third and final Delphi round, with 80-100% consensus on the tool’s signalling questions, including those with minor adjustments to the wording. We also established a consensus of >70% that the overall ROB judgment should match the most severe ROB judgment on an item, unless appraisers can provide justifications to rate the overall ROB lower (eg, many concerns on many items) or higher (eg, concern seems not to have an important influence on overall ROB). For example, if multiple subdomains were rated as very serious, the final judgment could be rated as extremely serious (supplement S7).
Risk-of-bias subdomains
ROBVALU includes seven key signalling questions across four subdomains: selection of participants into the study, completeness of data, measurement instrument, and data analysis (table 2).
Subdomains and considerations in the Risk of Bias in Values and Utilities (ROBVALU) tool
Selection of participants into the study
Precise research questions include a clear definition of the target population. The study population of any empirical study must be representative for this target population, and is therefore, a critical component because bias in the selection will lead to biased estimates of the values people place on outcomes in the target population.2 When assessing selection bias, users should consider the study’s sampling strategy, in particular if the achieved sample population deviates from the intended sample population,2 because this might lead to biased estimates for the study’s population of interest owing to threats to internal validity. If the achieved sample population does not deviate from the intended sample population but differs from the population researchers intend to extrapolate the results to, this difference will result in a lack of generalisability. We refer to this lack of generalisability as indirectness, which encompasses applicability and external validity. The ROBVALU tool is not intended to deal with indirectness, a different domain in assessing the certainty of a body of evidence according to GRADE, but we are developing a tool that is specific to indirectness separately.
Completeness of data
When judging completeness of data, reviewers need to consider the response rate of the study population, the attrition rate if follow-up was involved, and the differential responders compared with non-responders.2 High response rates and low proportion of loss to follow-up are clearly preferable, and a high proportion of non-response or dropout rates could be problematic.2 Participants providing responses could plausibly differ from those who do not, and researchers should consider that results coming only from those participants who responded or completed follow-up might be misleading.2
Measurement instrument
Reliable and valid instruments should be used to measure the relative importance of outcomes in values, preferences, and utility studies.2 Using unreliable or poorly validated instruments can result in biased measurements of the outcome. Similarly, utility values for specific health states based on instruments not sufficiently validated that are used as input parameters for decision analytical models can result in biased estimates, such as quality adjusted life years derived from state transition models.3132 Researchers conducting primary empirical studies should provide information regarding the measurement properties of their chosen instrument.2
Researchers should also demonstrate that the instrument has been used correctly and in a consistent manner across all participants in a study. For example, if the standard gamble is to be administered by an interviewer, buta subset of participants used self-administration, this could result in biased utility estimates that could be due to systematic differences between the two groups. In addition, an optimal representation of the outcome or health state should be presented or described in a way that accurately reflects the attribute the researchers intended to measure. This information could include a detailed explanation of how the outcome defines the experience, the probability of the outcome, durations, and possible consequences. Finally, researchers should evaluate whether participants had a proper understanding of the instrument to complete the tasks.
Data analysis
Studies should explore heterogeneity in values when appropriate and present results for the different subgroups. The data analysis plan and exploration of heterogeneity should be outlined a priori before collection of data. A causal framework that helps delineate health state and outcome interactions with possible confounding factors will help make assumptions explicit. If heterogeneity is found, the evaluator needs to consider whether the adjustment, stratification, or model selection used in the study reporting on values was appropriate.2 Adjusting for important confounding factors (such as age if it is associated with the intervention and influences the estimated values) or reporting values in a stratified manner reduces biased estimates of the value placed on an outcome. In addition, self-inflicted biases, including selection bias or immortal time bias should be controlled for appropriately using modern causal inference methods (eg, target trial emulation or g methods for time varying confounding).33
ROBVALU tool application
The assessment of ROB in studies evaluating the value people place on outcomes follows seven steps:
-
Specify the research or review question.
-
Specify the outcome being assessed.
-
Identify the sampling frame, the response rate and/or attrition rate, the measurement instrument used, and the data analysis plan.
-
Answer the signalling questions of the four subdomains.
-
Make a judgment if the four subdomains have important ROB concerns.
-
Formulate a ROB judgment for the four subdomains.
-
Formulate an overall ROB judgment for the study outcome being assessed.
The ROBVALU tool (supplement S8) provides users with space to record vital information of the study being assessed, and signalling questions to all four subdomains that must be answered. We validated a 4 point Likert-type scale (yes, probably yes, probably no, no) to respond to the individual signalling questions (items). When rating individual signalling questions, we suggest following the flowchart in figure 2 for consistent answers between raters. In each subdomain, the tool asks to specify how important the ROB issue is on a 4 point Likert-type scale (yes, probably yes, probably no, no), and how serious the overall ROB issue is on a 4 point Likert-type scale (not serious, serious, very serious, extremely serious). Responses to the signalling questions should provide the basis for the subdomain level judgment, of how important and how serious the ROB issues are in the study. Raters should provide a rationale for the response as free text, to justify their judgments. We suggest that the final judgment for each subdomain inversely correlates with the signalling question judgment. For example, in the measurement instrument subdomain, if the answer to “Was the instrument administered in the intended way?” was “No,” then the answer to “Are there important risk of bias issues concerning the measurement instruments?” should be “Yes.” If raters believe that the lowest signalling question judgment does not reflect the overall subdomain judgment, they might choose not to deem the results of the study at ROB for that subdomain, but they are asked to provide explanations for why they would not do this.
The global ROB judgment for a study corresponds to the lowest subdomain judgment (table 3), because any domain level bias will lower our confidence in the study results. If users do not believe that the lowest subdomain judgment reflects the global ROB judgment, they should provide a justification. For example, if a study has a low response rate resulting in very serious ROB domain judgment and the study results are comparable to better quality studies, a reviewer might consider that the subdomain judgment does not reflect the global ROB judgment. Box 1 presents an illustrative example of a completed assessment (supplement S9).
Response options for judgments on risk of bias at an overall study level, according to the Risk of Bias in Values and Utilities (ROBVALU) tool
Example application of the Risk of Bias in Values and Utilities (ROBVALU) tool to assess risk of bias in values assigned to exacerbation of chronic obstructive pulmonary disease34
In a study of 65 men and women with chronic obstructive pulmonary disease, researchers assessed the utility value that participants placed on an exacerbation, at seven study sites in the US when they visited an outpatient clinic within 48 hours of symptom onset.34 Eligible participants were at least 40 years old and were current or former smokers with a history of at least 10 pack years. Of 65 participants, 59 completed the study, three were lost to follow-up, and three were ineligible. Utility values were measured using the EQ-5D.
An assessment using the ROBVALU tool revealed the following (supplement 9):
-
Selection of participants into the study would likely lead to risk of bias.
-
Exacerbations that required hospital admission were considered severe and were excluded from this study and might importantly bias the estimates. Thus, the population was deemed to be probably not representative of the intended population.
-
-
Completeness of data was present:
-
Measurement instrument caused some concern about risk of bias:
-
It was not clear whether the instrument was used in a valid and reliable manner, but it was applied in the intended way using a valid representation of the outcome. Patients also appeared to show an understanding of the instrument that was used and did not encounter difficulties, but this was not reported.
-
-
Data analysis did not cause concern for risk of bias:
ROBVALU assessment
Overall risk of bias was deemed serious because of issues related to the selection of participants into the study and the way the measurement instrument was used.
Discussion
We have developed and validated the ROBVALU tool, a new instrument to assess ROB in studies measuring the value, utility, or relative importance that people place on health outcome. We followed a sequential mixed methods approach, by first adapting the signalling questions from the GRADE guidance for judging ROB across studies. ROBVALU differs from existing GRADE guidance by specifically assessing ROB in individual studies as opposed to across studies.2 We iteratively revised the tool with our core group and an advisory group. The final draft tool contains 15 items in four subdomains: selection of participants, completeness of data, measurement instrument, and data analysis. We conducted a validation exercise with 15 participants that showed good reliability. Additional refinement using a modified Delphi process established construct validity on the final content of the tool.
Strengths and limitations
Assessing ROB is an essential step to assess the overall certainty of the evidence in a systematic review or health technology assessment and to develop a guideline. This assessment has often relied on adapting ROB tools not specifically designed for this type of research.26 However, the lack of validation could lead to unreliable certainty of the evidence assessments, both for single studies and for a body of evidence. By using ROBVALU, evaluators can incorporate the ROB assessment into their meta-analysis, such as performing a sensitivity analysis to evaluate how studies with higher ROB might affect the study’s conclusion or primary outcomes. An advantage of the ROBVALU tool is the use of standardised GRADE terminology and judgments to facilitate assessment when establishing the certainty of the evidence. The ROBVALU tool can also be used to assess ROB in all elicitation studies of values, utilities, and importance of outcomes that use discrete choice, ranking, indifference, and rating methods.35 Finally, the tool can be used in individual studies that use indirect methods to elicit people’s preferences, such as quality of life and EQ-5D scores.
This study and the derived tool also has several limitations. The new tool focuses on assessing values quantitively. For any given intervention, there is usually qualitative literature exploring what patients want to achieve and what they value (or not) from interventions; this information could be important for decision making. While some of the signalling questions might be used for qualitative studies, other signalling questions will not apply. Further exploration with qualitative studies should be performed to assess how ROBVALU can be adapted for that particular use, or whether a different tool is required. Furthermore, an exploratory factor analysis showed that one item in the tool had relatively poor fit (Was a valid representation of the outcome (health state) used?), but this poor fit could be due to the relatively small sample size. However, we retained this item because of feedback from the Delphi panel, who deemed it important. External validation of ROBVALU’s reliability by different users and on different studies will help refine the guidance and the tool.
Future implications
ROBVALU allows researchers to appraise individual studies reporting utilities, values, or the importance of outcomes for risk of bias. For example, in health technology assessments, the certainty of input variables from an individual study determines the certainty of outputs from decision analytical models (eg, cost utility and cost effectiveness analyses).3236 ROBVALU should also help with evaluating ROB as part of a systematic review, health technology assessment, or formal health guideline, to develop recommendations and make judgments across the overall body of this type of evidence (eg, assessing overall certainty of the evidence when following the GRADE approach).
Ethics statements
Ethical approval
This international study was designed and coordinated at McMaster University after approval by the Hamilton Integrated Research Ethics Board (project ID 5634), and interviews and meetings were conducted in person or over video conference. All participants provided informed consent.
Footnotes
-
Contributors: The authors are epidemiologists, statisticians, systematic reviewers, and health services researchers, many of whom are involved with methods research and GRADE. Development of ROBVALU was informed by GRADE guideline 19, previously published tools for assessing risk of bias in intervention studies, systematic reviews of available tools to assess risk of bias in values and preferences, and the authors’ experience of developing similar tools to assess risk of bias. All authors contributed to development of the ROBVALU tool and to writing associated guidance. SGK, YZ, JLB, and HJS designed the study and formed the core group. YZ, JLB, and HJS conceived of the project. HJS oversaw the project and is guarantor. SGK, YZ, TD, JLB, and HJS drafted the ROBVALU tool. JN, PAC, FX, and US formed the advisory group. SGK led working groups and conducted the semi-structured interviews. SGK and LM analysed the data. HP-H, GH, YZ, and PAC assessed studies. PAC, FX, BE, ZSP, VW, ALS, J-ET, JN, LK, and US participated in the Delphi process as voting members, and HJS, YZ, SGK, and JLB were non-voting members. SGK and HJS drafted the manuscript. YZ, JLB, and HJS obtained funding for the study. All authors reviewed and commented on drafts of the manuscript. The corresponding author attests that all listed authors meet authorship criteria and that no others meeting the criteria have been omitted.
-
Funding: The study was funded by the Canadian Institutes of Health Research (grant 401310 to HJS and JLB). The funders had no role in considering the study design or in the collection, analysis, interpretation of data, writing of the report, or decision to submit the article for publication.
-
Competing interests: All authors have completed the ICMJE uniform disclosure form at www.icmje.org/disclosure-of-interest/ and declare: support from the Canadian Institutes of Health Research for the submitted work; no financial relationships with any organisations that might have an interest in the submitted work in the previous three years; no other relationships or activities that could appear to have influenced the submitted work.
-
Provenance and peer review: Not commissioned; externally peer reviewed.